Preface
1. Purpose
The purpose of this book is to help you learn to implement and analyze evolutionary models of social interactions in finite populations. This goal may not be perfectly clear to you right now (and that is absolutely fine), so let us examine the main terms in the bold sentence above, one by one.
- By “social interactions” we mean interactions between individuals where the outcome for each individual depends not only on her own actions, but also on the actions of others. A simple example is the decision to drive on the left or on the right of a two-way road. Driving on your left is a good idea if everyone else also drives on their left, but not so good if everyone drives on their right. The interaction between a penalty kicker and the goal keeper is another example. A more complex example would be the decision to start a new collaboration with a partner or not and, if so, how much effort to put into the partnership. We will see in this book that game theory is a great framework to formalize and analyze this type of social interactions.
- By “evolutionary model” we mean a model where the individuals involved in the interaction may change their actions in time, according to some decision rule. An example of a decision rule could be: “Look at what other individuals are doing, identify who is doing best, and copy what she is doing (i.e., her action)”. If individuals update their actions in time, the distribution of actions in the population changes or evolves. Evolutionary models at their core are just dynamic models, i.e. models where there is some change in time. We will see in this book that evolutionary game theory is a great framework to formalize and analyze this type of dynamic models of social interactions.
- By “finite populations” we mean that there is a finite number of individuals in our models. You may be wondering: what else could it be? Well, the point is that many models in evolutionary game theory assume that the population is infinite. Normally, this assumption is made for mathematical tractability, i.e., for analytical convenience. Here we will not impose that assumption. Our object of study will always be a model of a population which, in principle, could exist in the real world.
- By “learn to implement evolutionary models“, we mean that we are going to learn how to code our evolutionary models in a programming language, using a computer. It is perfectly fine if you have never programmed before; actually, if that is the case, we believe that you are going to enjoy this book immensely. If, on the other hand, you have some programming experience, chances are that you will sail through many chapters of this book faster than you think. In this book we are going to use an agent-based (aka individual-based) approach, i.e. we are going to implement how individuals make their own decisions, how they individually change their actions in time, and we are going to see how they individually enjoy or suffer the consequences of their individual decisions. We will also be able to see how the distribution of actions in the population changes in time, according to the different assumptions that we wish to implement in our models.
- And finally, by “learn to analyze evolutionary models” we mean that we will do our very best to understand the dynamics of our models, i.e. we are going to find out why we observe what we observe, and try to derive conclusions about the logical implications of the assumptions we implement in our models.
The following paragraphs explain why the two skills we are going to learn with this book –i.e. model implementation and analysis– are key for scientific modeling.
Model implementation
To use a scientific model rigorously, it is important to be fully aware of all the assumptions embedded in it, and also of the various alternative assumptions that could have been chosen. If we don’t understand all the details of a model, we run the risk of over-extrapolating its scope and of drawing unsound conclusions. A great way to understand a model in depth is to implement it in computer code following an agent-based approach. We believe this is true regardless of whether the model is currently expressed in natural language (and may even exist only in your mind) or, alternatively, it is written down in mathematical language (e.g. using equations).
Coding a model expressed in natural language is very useful because it ensures that the model is both unambiguous and completely specified. A computer implementation of a model is necessarily unambiguous because the language used to code it (i.e. the programming language) is formal, so it does not allow ambiguities to infiltrate; symbols and instructions used in programming languages have always the same meaning regardless of context. A computer implementation of a model must also be completely specified before it can be run, since computers do not make assumptions by themselves. Thus, to execute a model in a computer, there cannot be any loose ends in the description of the model. This contrasts with models expressed in natural language, where it is easy to leave aspects of the model partially unspecified –often unintentionally–, since our brains are particularly good at using context to unconsciously fill the details. If we use natural language to describe a model, the audience may understand something slightly different from what we mean to communicate, and results may be driven by assumptions that we have not made explicit. By contrast, computers need all assumptions to be spelt out, and this requirement makes the process of scientific modeling more sound and rigorous.
If a model is written in the language of mathematics, the problems outlined in the paragraph above are no longer an issue. Thus, is it really worth implementing a mathematical model in computer code following an agent-based approach? We believe that, in many cases, it certainly is. The reason is that many mathematical models contain assumptions that are desirable for analytical tractability, but which also weaken the link between the model and the real world. These assumptions made for analytical convenience tend to elevate the mathematical model to a higher level of abstraction and aggregation. By contrast, the agent-based approach has the advantage of forcing the programmer to implement the microfoundations of a model explicitly, considering each individual agent as a separate entity. This requirement helps the modeler be aware of all the assumptions that are made in the mathematical model, and it also allows for an assessment of their significance.
Model analysis
Once the model is implemented, the way to fully understand it is to analyze it. In this book, we will see several techniques that are useful to analyze finite-population evolutionary models, including Markov chains, Monte Carlo simulations, mean dynamics, stochastic stability analyses, diffusion approximations and pair approximations. For each of these techniques, we give a brief introduction, illustrate its usefulness with concrete examples, and provide references for the interested reader to learn more about it.
2. Structure of the book and potential courses
In this section we present an overview of the whole book and suggest different paths that readers may want to follow, depending on their particular interests and the time they want to spend on the book.
The book is divided into five parts, numbered in roman numerals:
- Part I. Introduction
- Part II. Our first agent-based evolutionary model
- Part III. Spatial interactions on a grid
- Part IV. Games on networks
- Part V. Agent-based models vs ODE models
These parts are largely independent and self-contained, except for Parts IV and V, which build on Part II. Each part contains several chapters. Each chapter corresponds to one webpage in the webbook.
Part I is somewhat special in the sense that it contains introductions to Evolutionary Game Theory (chapter I-2), Agent-based Modeling (chapter I-3), NetLogo (chapter I-4) and programming in NetLogo (chapter I-5). These introductions are self-contained and independent, so the reader who is already familiar with some of these topics can perfectly skip the corresponding chapters.
The modular design of the book allows for the configuration of different courses. The following lists provide some examples.
Courses on Agent-Based Modeling (ABM):
- Basic course on ABM: Chapters I-3, I-4 and I-5, plus Part II. Optional: chapter I-2.
- Course on ABM, including spatial interactions: Parts I, II and III.
- Course on ABM, including networks: Parts I, II and IV.
- Advanced course on ABM: Parts I, II, III and IV.
Courses on Evolutionary Game Theory (EGT):
- Basic course on EGT: Parts I and II.
- Intermediate course on EGT: Parts I, II and V.
- Course on EGT, including spatial interactions: Parts I, II, III and V.
- Course on EGT, including networks: Parts I, II, IV and V.
- Advanced course on EGT: The whole book.
The book contains more than 100 exercises with detailed solutions. The exercises are proposed at the end of most chapters, in a section titled “Exercises“. The solutions for the exercises proposed in each Part are included in the last chapter of the corresponding Part, which is titled “Answers to exercises“. These chapters are only present in the online webbook, and they are protected with a password. If you are not a student following a course based on this book, feel free to contact us to obtain access to these solutions.
3. Why NetLogo?
This book uses a programming language called NetLogo (Wilensky, 1999) to implement several models. In chapter I-4 we explain many of the features that make NetLogo our language of choice. Nonetheless, the main reason we chose NetLogo is that it is remarkably close to natural language. As a matter of fact, we believe that NetLogo language can perfectly be used as pseudo-code to communicate algorithms. This means that, in theory, a proficient programmer could use this book to implement the proposed models in her language of choice, rather than in NetLogo.
Given how easy it is to understand NetLogo code, admittedly, we have been very sparing in commenting our code. The reader may want to comment her own code much more than we do, and we definitely recommend this good practice. We did not comment our code much because we wanted to provide code as clean as possible, and also because comments are fairly personal; everyone has her own personal difficulties when programming and understanding code.
4. One book, many formats
This book has been written and formatted following a hands-on approach. It contains several (internal and external) hyperlinks, images, videos, code snippets, links to NetLogo programs, etc. The book comes in different formats:
- Webbook: This is the online version of the book. We believe this is the most convenient format for most readers. Its only drawback is that it requires access to the internet.
- Digital PDF: This a pdf document that you can download and use online or offline. This format preserves all the hyperlinks of the webbook, and these hyperlinks use the same colored fonts as in the webbook. Naturally, you will need to be online to follow the external links. Also, note that in this format all the videos have been replaced with hyperlinks, so you will need to be online to watch the videos.
- Print PDF: This is a pdf document that has no hyperlinks (nor videos). The hyperlinked (colored) words in the Digital PDF are not colored in this format, so readers who want to print the pdf may prefer this version to the Digital PDF.
- EPUB: This is an e-book file that can be read by most e-book readers. All images and videos are contained inside this file, so they can be seen without access to the internet.
In addition to these formats, we also provide Common Cartridge Files. These files contain web links to the different chapters of the book, and are useful to import parts of the book into Learning Management Systems such as Canvas, Moodle or Blackboard.
5. What about the programming and the math?
We are hopeful and confident that if you go through the whole text, implement the proposed models, and try to do some of the exercises included at the end of most chapters, you will master the art of implementing and analyzing agent-based evolutionary dynamics, using both computer simulation and mathematical analysis.
Nonetheless, we also have in mind two other types of less committed readers:
- If you are interested in learning how to analyze finite-population evolutionary models, and in their relation to other models in Evolutionary Game Theory, but you do not wish to program, then you should skip all the sections preceded by the NetLogo icon
. These are the sections with a strong programming component.
- If you want to become proficient in coding agent-based models, but you are not interested in learning how to analyze these models, please do reconsider your preference. If your preference persists after careful reflection, you may want to skip the chapters titled “Analysis of these models” (which you will find at the end of Parts II, III and IV) and also chapter V-3. These are the chapters with a strong mathematical component.
Our hope is that, regardless of the discipline you are coming from, your background and your preferences, this book will help you learn new and exciting ways of understanding evolutionary systems.
6. History of the book and acknowledgments
The first steps of this book date back to 2016. That year, we —Segis and Luis— were awarded a grant to visit world-renowned Professor Bill Sandholm at the University of Wisconsin–Madison for three months. Having read his work, we had extremely high expectations for our in-person meeting with Bill.
Bill exceeded all our expectations by orders of magnitude in every conceivable way. Every minute spent with him was full of wisdom, generosity, awe, admiration, and joy. Every day spent working with him was worth a year on our own. Meeting Bill was, without a doubt, the decisive moment in our scientific careers.
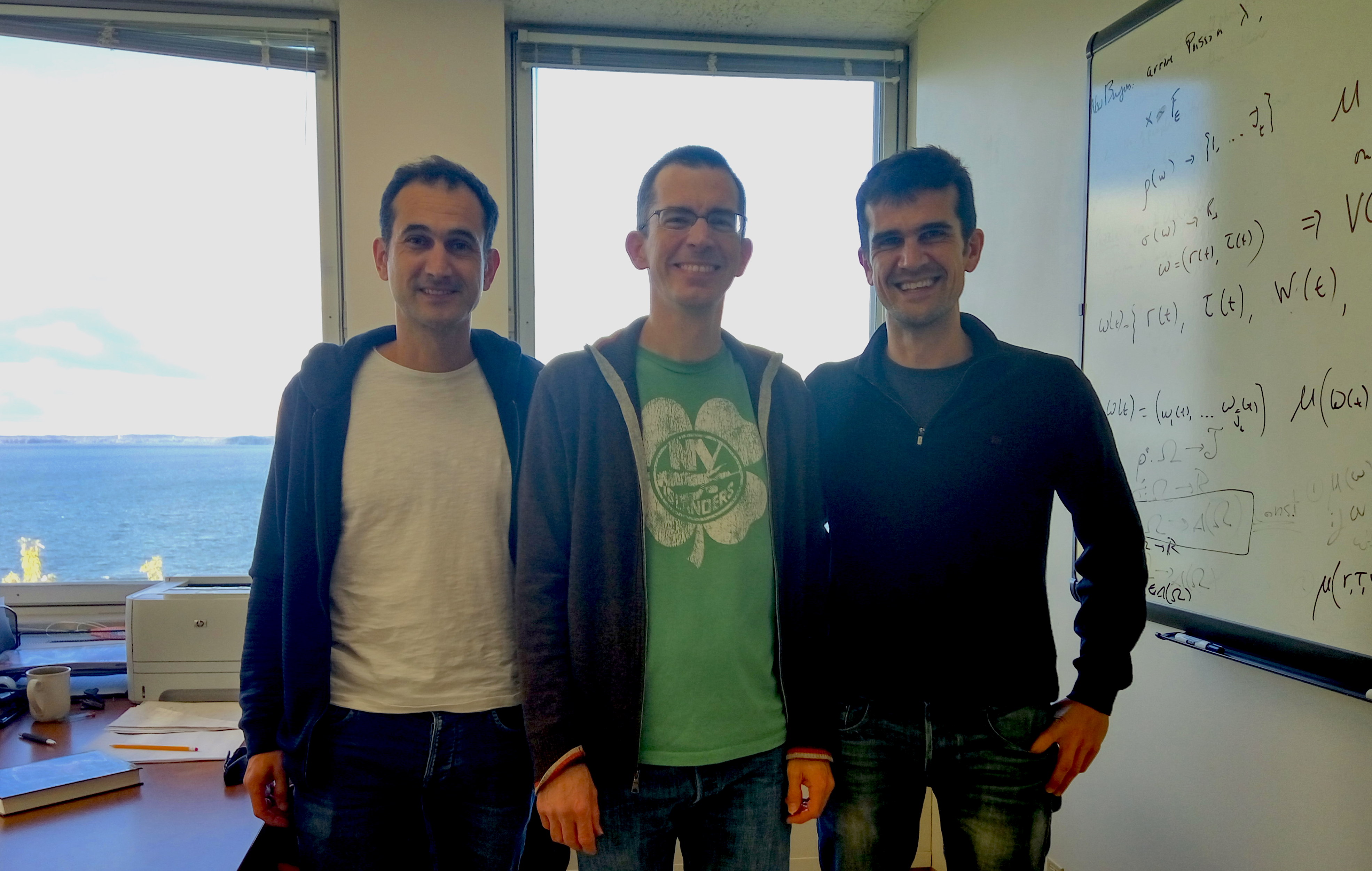
The primary goal of our visit was to design this book, and we hoped that Bill would review our proposal and provide some feedback. Not only did he fulfill our request with the greatest interest and professionalism, but he also offered his assistance in every way he could. Specifically, he introduced us to Steel Wagstaff, an extremely competent and inspiring instructional technology consultant who dedicates his life to doing wonderful things for wonderful reasons. Steel suggested we could use Pressbooks to publish this book, set everything up for us to begin writing, and instilled in us his passion for developing Open Educational Resources. Since then, Steel has been a constant source of motivation and support. This book would never have existed in its present form had we not met Steel.
After working with Bill for a couple of months, he honored us by accepting our invitation to contribute to the book as an author. Then, over the following years, we had the great privilege of collaborating with Bill on various projects, including this book. During those wonderful years, our admiration for Bill only grew, and every day we felt incredibly fortunate to have the opportunity to work alongside such an amazing scholar.
Then, on a fateful summer day in 2020, we received the devastating news that our dear mentor and friend had left this world.
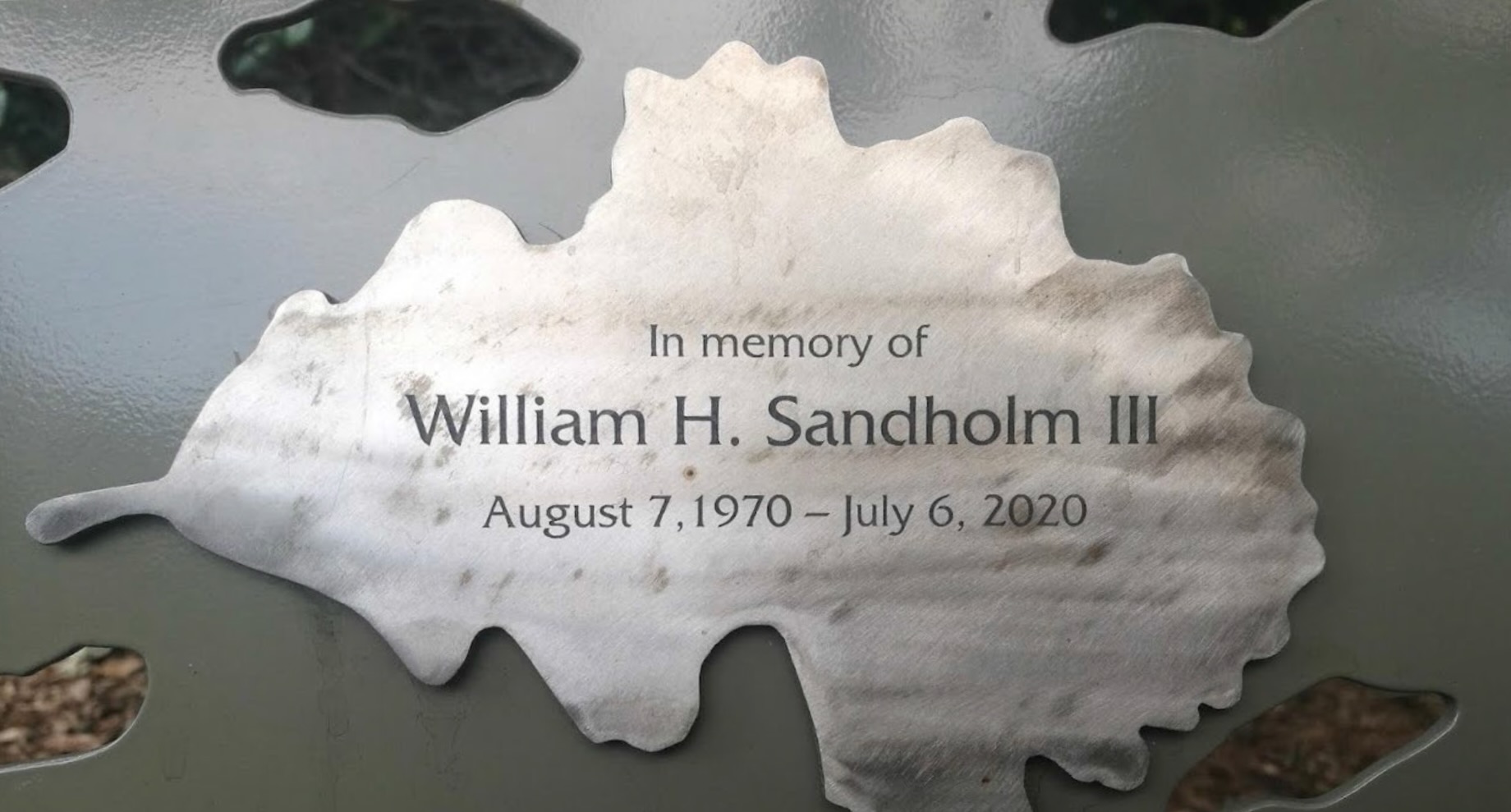
After Bill’s passing, it took us a long time to muster the courage and energy to continue working on a book that we had begun with him. Eventually, we found the strength to pull ourselves together and finish the book at the University of British Columbia, largely thanks to the wonderful support and care of Christoph and Claudia Hauert.
Our greatest hope now is that Bill would feel proud to see his name on the book’s cover. If you find anything unclear or mistaken in this book, it is certainly our fault; if you discover something beautiful or inspiring, Bill is likely to be behind. We profoundly miss Bill’s extraordinary intelligence, kindness, playful spirit, and commitment to scientific rigor. But, most importantly, when we think of him, we are filled with immense gratitude. We are truly thankful for the chance life afforded us to learn so much from such an incredible and remarkable friend.
This book could not have been finished without the help of many others. We are deeply indebted to our home universities —the University of Burgos (UBU) and the University of Valladolid (UVa)— for allowing us to travel occasionally to visit other institutions and learn from the best. We have worked on this book while at the University of Wisconsin–Madison (UW–Madison), the University of California, Santa Barbara (UCSB), and the University of British Columbia (UBC). We could not have made and enjoyed these trips so much without the wonderful support of Jose M. Galán, Nacho Santos, Nacho Fontaneda, Juan José Lavios, and José M. Cámara (UBU), the EII section of the Department of Business Organization (UVa), Marzena Rostek, Daniel Quint, Marek Weretka, and Ananth Seshadri (UW–Madison), Jason Marden (UCSB), Daniel Coombs and, most especially, Christoph Hauert (UBC). We would also like to gratefully acknowledge the financial support from the Spanish State Research Agency, the Fulbright Program, the Spanish Ministry of Science, Innovation and Universities, and the European Regional Development Fund.
Academically, many scholars have profoundly influenced and enhanced our understanding of agent-based evolutionary dynamics, and we are immensely grateful to them. In particular, we acknowledge Fernando Vega-Redondo, Christoph Hauert, José M. Galán, Nick Gotts, Gary Polhill, Bruce Edmonds, Jörgen W. Weibull, Nacho Santos, Francisco Fatás-Villafranca, Koen Frenken, Isabel Almudí, Doina Olaru, Ryan McAllister, and Cesáreo Hernández for their pivotal roles in shaping our academic careers.
Finally, we would like to acknowledge our greatest debt. Over the past eight years, our beloved families have made tremendous sacrifices so we could write this book. Carmen and Gemma had to leave their jobs in Spain three times. Luis left his friends behind and followed us into the unknown, Daniela changed schools five times and Alejandra had to learn to walk on a transatlantic flight. They all had to endure countless nights sleeping on the floor of unfurnished student flats adorned with camping chairs. Most families we know would not have survived the ordeals our families have been through to support us. Only two families we know can do it with a smile on their face and remember all of it as a great adventure. We cannot thank them enough for that.